
We explore different variations of LSTM, CNN, and Attention-based neural networks for comparison. CNNs are also pretty popular for sentence classification tasks where a fixed length padded word vector sequence is passed through the CNNs. A more sophisticated approach is to pass the sequence of word vectors through LSTM and use final hidden state representation for classification. Naive bag of words approach is to average the word embeddings and then use a linear classifier or feed-forward neural network to classify the resulting sentence embedding. The embedding matrix is used as an input to deep learning models. In this work, we use FastText word vectors, pre-trained on Hindi corpus. Usage of pre-trained vectors have shown to give superior results and are thus the de-facto method to represent word tokens in all NLP models. This gives some useful semantic properties to low dimensional word vectors. The similarity of the word vectors are correlated to the semantic similarity between actual words. A very common approach is to learn these distributed vectorial representations using unsupervised learning techniques like word2vec The sequence of vectors is processed by classification algorithms. Instead of passing the raw character tokens, each word is mapped to a numerical vector. Each sentence split into a sequence of word tokens and passed to classification algorithms. In this work, we consider sentence-level classification tasks. They work at the sentence level, paragraph level or document level.

Even the multi-lingual datasets like XNLI used for evaluation of natural language inference tasks is based on the translation.Ĭurrent text classification algorithms are mainly based on CNNs and RNNs. We believe this is the right time to evaluate the translated data sets. Self attention-based models like transformer have resulted in best in class results for translation tasks. This is indeed great times for translation systems. In order to create Hindi dataset in Devanagari script, standard datasets like TREC, SST were translated using Google translate. This work will help in the selection of right models and provide a suitable benchmark for further research in Hindi text classification tasks. Increase in the robustness of translation and transliteration systems have also contributed to the rise of NLP systems for Hindi text. Service providers, e-commerce industries are now targeting local languages to improve their visibility. Moreover, there has been a substantial rise in Hindi language digital content in recent years.
#SUNDERKAND IN HINDI TEXT FREE#
However, Hindi is morphologically rich and relatively free word order language so we investigate the performance of different models on Hindi text classification task. While the most important reason for this is unavailability of large training data another reason is generalizability of deep learning architectures to different languages. There have been very few text classification works in literature focusing on the resource-constrained Hindi language. It is an integral component of conversational systems for intent detection. It finds application in sentiment analysis, spam detection, email classification, and document classification to name a few. Text classification is the most widely used NLP task. The focus of our work is text classification of Hindi language. Machine learning approaches have been used to achieve state of the art results on NLP tasks like text classification, machine translation, question answering, text summarization, text ranking, relation classification, and others. Ability to learn from the data have made the machine learning system powerful enough to process any type of unstructured text. NLP in the context of deep learning has become very popular because of its ability to handle text which is far from being grammatically correct. The language can either be represented in terms of text or speech. Natural language processing represents computational techniques used for processing human language. The paper also serves as a tutorial for popular textĬlassification techniques. On BERT and LASER are also compared to evaluate their effectiveness for the Multilingual pre-trained sentence embeddings based Work, we used translated versions of English data-sets to evaluate models based
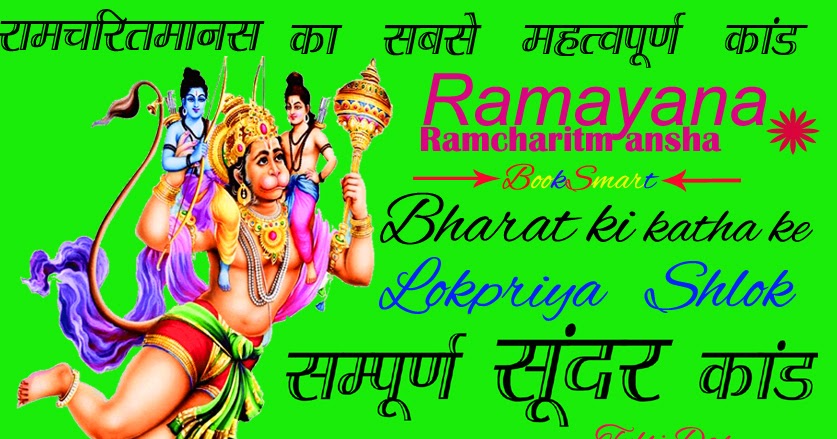
Script has been limited due to the absence of large labeled corpus. Morphologically rich and low resource Hindi language written in Devanagari In this work, we survey a host of deep learningĪrchitectures for text classification tasks. LSTM, and very recent Transformer have been used to achieve state of the art

Different deep learning architectures like CNN, Text processing has revolutionized the techniques for text processing andĪchieved remarkable results. Natural Language Processing (NLP) and especially natural language textĪnalysis have seen great advances in recent times.
